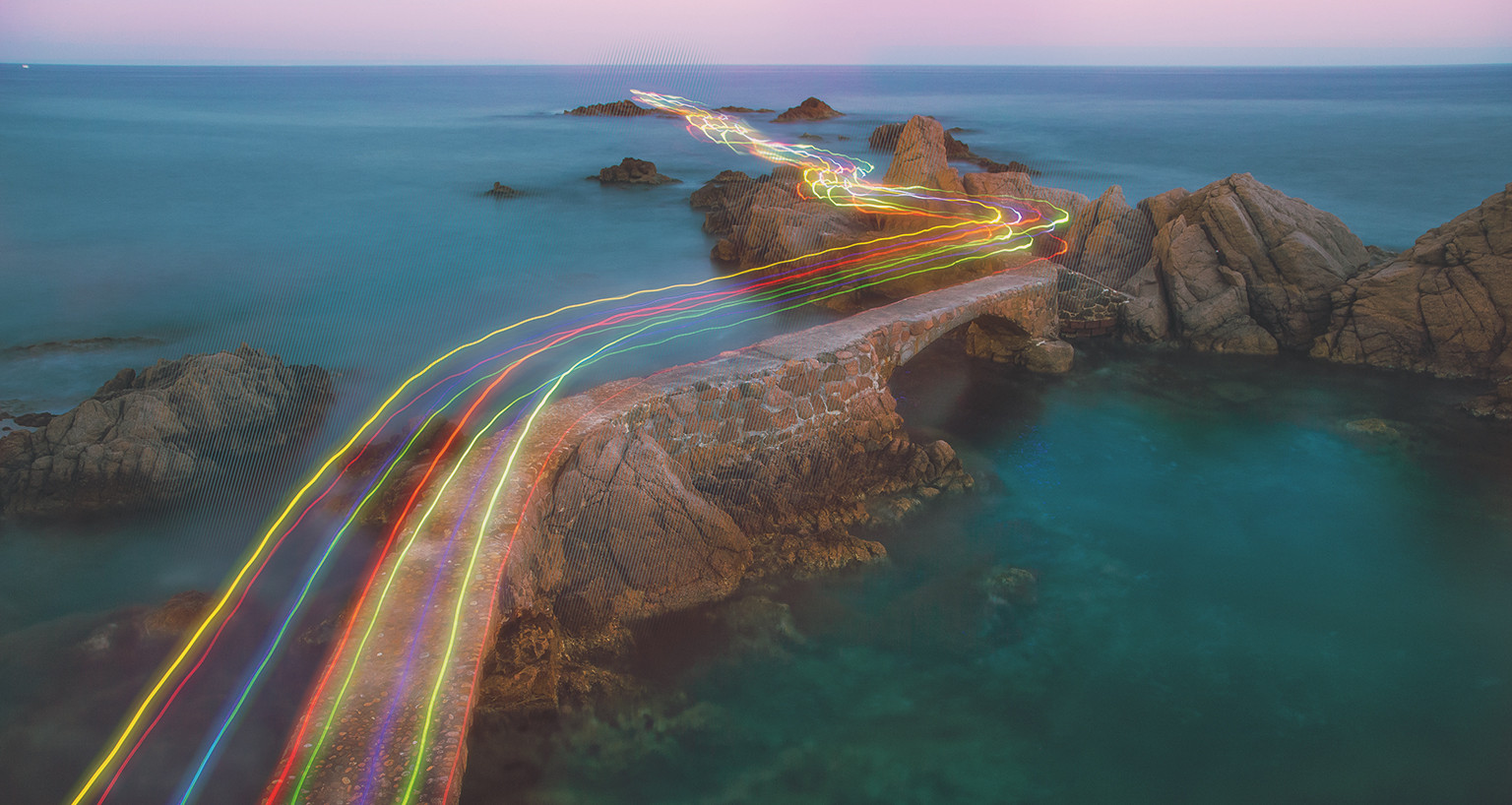
May 21, 2025 // Hannah Kuck
From Vision to Obligation: Banks and PSPs on the Road to Instant Payment
The new EU regulation makes SEPA Instant Payments mandatory from 2025. The countdown to implementation is now beginning for banks and payment service providers. What is required by regulation - and how fraud risks can be managed in real time.