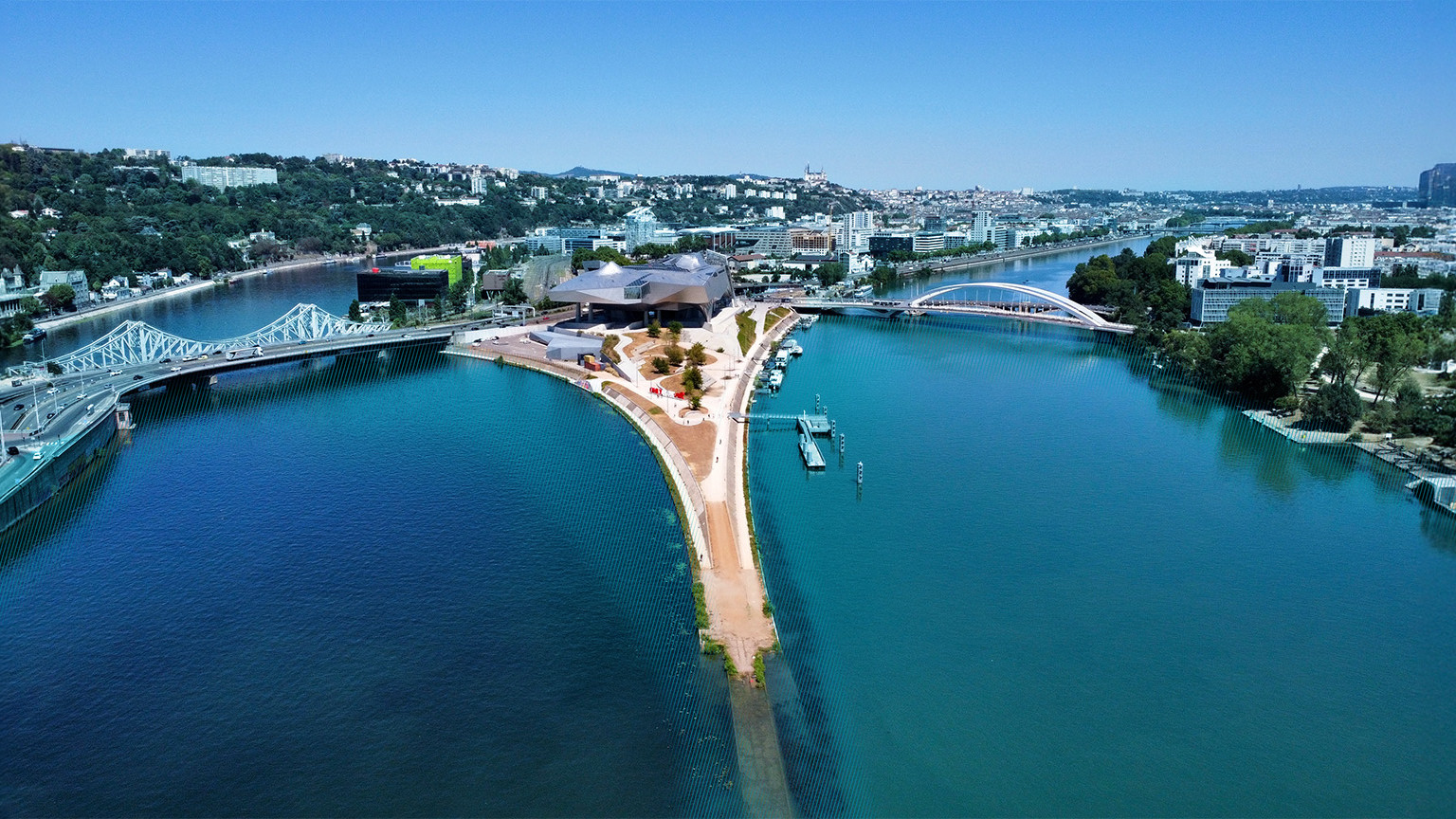
Jul 14, 2025 // Katrin Schlüter
FRAML Blog – Rewards, Risks, and Realities of Integration
FRAML is more than just a buzzword: it’s a shift in how financial institutions approach fraud and AML. This blog unpacks what’s driving the trend, where the risks lie, and how it can be made to work in practice.